​Ho-Yin Mak
Associate Professor, McDonough School of Business, Georgetown University​
“A great city is not to be confounded with a populous one.” -- Aristotle
With rapid urbanization, our cities are growing in size and complexity. This calls for innovative smart city approaches to ensure efficient, equitable and sustainable management of urban services and infrastructure. In business, this movement has engendered the emergence of new industries and redefined competitive priorities in existing ones. Cases in point include the rise of clean technology in energy and transportation, and analytics-driven urban logistics for online retail, all of which have propelled disruptive innovations in their respective sectors.
My recent research in smart city operations management aims to address how firms sustain or redefine their competitive edges under these paradigm shifts, via the pursuit of managerial insights into novel research questions, as well as analytics solutions that support practical decisions. Below, I outline some of my recent and ongoing research in three aspects of smart city OM, namely, sustainable transportation, urban supply chains, and the sharing economy.
Sustainable Transportation: Electric Vehicles
Transportation accounts for 15-20% of global greenhouse gas emissions. As a key technology to cleaning the transportation sector, electric vehicles (EVs) have recently received much press coverage, as both the UK and France have announced to completely ban the sale of internal combustion engine vehicles by 2040, forcing automakers to electrify their product lineups. Despite the long-term potential, however, the development of EV markets at present is relatively nascent and being hampered by a few major roadblocks.
​
Two of the well-cited barriers against mass adoption of EVs are the range and resale anxieties. In paper [1], we employ a game theoretic approach to analyze the automakers' optimal business model design in the presence of these anxieties. We find that, although anxieties cause car buyers to undervalue EVs' performance (range) and resale values, such consumer underconfidence is not always detrimental to the long-term development of EVs. In particular, they may pressure automakers to over-invest in charging infrastructure, which benefits the market in the long run. Overall, the business model of combining range extension (by offering enhanced charging infrastructure) and selling EVs with their batteries (rather than leasing them) is typically the most conducive to mass adoption.
​
​Aiming to eliminate range anxiety, automakers appear to be engaging in an arms race in bringing longer-range EVs to the market, taking advantage of production economies of scale for lithium ion batteries. This year, Tesla and GM have launched EVs with over 230 miles of range (vs. ~100 miles for earlier mass market models) at relatively affordable prices (~$30,000 after incentives). Other automakers, such as Nissan, have taken a more cautious approach to making extended-range EVs – the newly announced Nissan Leaf model has a practical range of 150 miles. Considering that the daily driving needs for most people fall comfortably within the latter figure, it remains to be seen how much consumers value the extra peace of mind provided by additional range of the former. In working paper [2], we take the first step in addressing this question by empirically studying consumers' preference for EV range, based on a quasi-experiment in which a car sharing company converted its fleet from EVs to gasoline cars. Based on this unique dataset, we estimate the effects of a car's fuel type (electric vs. gasoline) and fuel/battery level (range) on its attractiveness to car sharing customers on a trip-by-trip basis.
​
​
​
​
​
​
​
​
​
​
​
​
​
​
Besides upgrading EV batteries, another crucial strategy to mitigate range anxiety is to deploy enhanced charging infrastructure, such as quick charging and battery switching stations. Considering the nascent development of EV markets, infrastructure planners face the problem of "little" (rather than "big") data: long-term decisions must be made given only limited data on travel patterns of EVs. In paper [3], we develop distributionally-robust optimization models for this infrastructure planning problem, taking into account inherent uncertainty and ambiguity in key travel pattern parameters. In working paper [4], we further refine these planning models to account for drivers' uncertain preferences for alternative recharging locations, by employing the persistency theory in robust optimization.
​
​
​
​
​
​
​
​
​
​
​
​
​
​
​
​[1] M.K. Lim, H.Y. Mak and Y. Rong (2015). Toward Mass Adoption of Electric Vehicles – Impacts of the Range and Resale Anxieties. Manufacturing & Service Operations Management, 17(1), 101-119.
​
​[2] S.W. Kim, H.Y. Mak, M. Olivares and Y. Rong. Empirical Investigation of Range Anxiety for Electric Vehicle Adoption. In Preparation.
​
[3] H.Y. Mak, Y. Rong and Z.J.M. Shen (2013). Infrastructure Planning for Electric Vehicles with Battery Swapping. Management Science, 59(7), 1557-1575.
​
[4] Y. Li, G. Ma and H.Y. Mak. Incorporating Drivers’ Preferences in Electric Vehicle Infrastructure Planning – A Conic Optimization Approach. Working Paper.
​
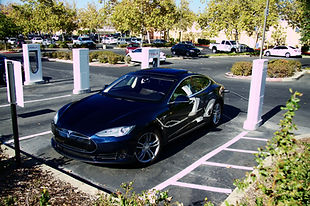
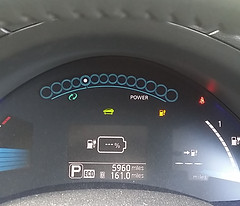
Range anxiety: concern over running out of battery [Source: my very own electric car almost running out of battery]
Charging infrastructure: where to locate when travel pattern data is limited? [Credit: Michael Hicks/cc-by-2.0]

Chevy Bolt: How much do consumers value its 238-mile range? [Credit: William Oliver/cc-by-2.0]
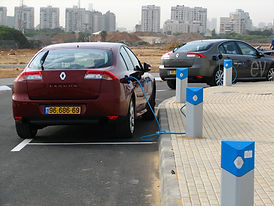
Better Place, whose infrastructure network proved too expensive as the company went bankrupt [Credit: User:Bardak/CC BY-SA 3.0]
Urban Supply Chain Management
Online retail in urban markets is undergoing a complete transformation. Faced with intensifying competition, fulfillment response times have become a competitive priority for mega-players such as WalMart and Amazon, in the key battleground of same-day deliveries. Omnichannel retailers (e.g., Walmart) hold the natural advantage of having conveniently-located physical stores to fulfill orders from. To counter this, Amazon, an online-only player, has substantially invested in bringing its fulfillment network closer to markets by locating smaller delivery stations and Prime Now hubs in major urban markets.
​
In terms of network design, deploying dense fulfillment networks appears to go against the conventional scale-based strategy in online retail, which suggests centralization of demand at a small number of large-scale warehouses to achieve economies of scale. In paper [5], we investigate the role of proximity, i.e., how close fulfillment facilities should be from customers, as a design feature in supply chain networks. We find that proximity interacts closely with the concept of agility, i.e., how quickly and flexibly the network can adapt its operations in response to changes in demand. In particular, we find that the scale-based network design strategy fares well for firms equipped with less agility, while more agile firms, such as those that has integrated their operations with real-time data analytics like Amazon, can benefit more from densely deployed fulfillment networks. In paper [6], we explore the role of agility in supply chain design, planning and operations and its implementation through advanced analytics.
Once the fulfillment network is in place, the key operational bottleneck is last-mile urban delivery. The innovative operations model of crowdshipping has been proposed as one possible solution. In working paper [7], we study the mode of crowdshipping where the omnichannel retailer enlists in-store shoppers to help deliver online orders for a fee, motivated by a recent experiment by WalMart. We focus on the implications of such a strategy on the marketing-operations interface: On the one hand, crowdshipping offers retailers the opportunity to enhance operations efficiency in last-mile delivery by saving on fleet and staff costs. On the other hand, crowdshipping alters the market segmentation between the online and offline channels, as it allows the retailer to cross-subsidize in-store shoppers (deliverers) using delivery fees from online shoppers. Our analysis reveals that, while crowdshipping benefits the retailer, whether it improves consumer surplus depends on how deliverers are compensated. Interestingly, compensating them a premium in addition to their incurred costs could undermine consumer surplus, compared with the case of simple cost-based reimbursements (or even the status quo without crowdshipping), despite the fact that each individual deliverer is better compensated.
[5] M.K. Lim, H.Y. Mak and Z.J.M. Shen (2016). Proximity and Agility Considerations in Supply Chain Design. Management Science, 63(4), 1026-1041.
​
[6] J. Acimovic, M.K. Lim and H.Y. Mak (2018). Moving Beyond the Speed-Price Trade-off. To appear in MIT Sloan Management Review.
​
[7] H.Y. Mak. Peer-to-Peer Crowdshipping as an Omnichannel Retail Strategy. Working Paper.
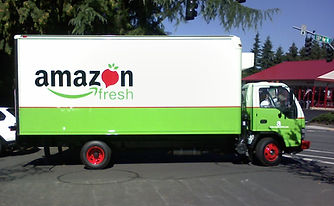
Retailers need smart supply chain strategies to compete effectively in the same-day delivery business [Credit: Jeff Sandquist/CC BY 2.0]

Could crowdshipping help solve the last-mile delivery puzzle?
Sharing Economy
One aim of the smart city development is to improve efficiency of resource utilization. With the aid of smart technologies, on-demand sharing platforms have become prevalent for various commodities and resources. In transportation, car sharing has been advocated by many as a means to reduce car ownership. The typical argument goes like this: A car is idle more than 90% of its lifetime on average. Therefore, individual ownership is wasteful and shared ownership is more efficient. This is an over-simplifying argument, as it overlooks the potential temporal (everyone needs the car at the same time) and spatial (the car is parked at an inconvenient location for other users) mismatch of supply and demand. Thus, the viability of car sharing critically hinges on addressing the key question of how supply and demand can be matched temporally and spatially.
Car sharing firms Car2go and Drivenow/Reachnow have been gaining traction with their on-demand, free-floating model of car sharing. Unlike conventional station-based car sharing models (e.g., ZipCar), customers are free to start and end their rental trips anywhere within a defined service region in the city, with or without advance reservations. In paper [8], we study the key planning question in this novel business model, namely, how to design the service region to offer extensive geographical coverage at competitive costs. In the free-floating model, demand is spatially unbalanced, due to a high proportion of one-way trips. To effectively match fleet supply with time-varying demand, the service region must be carefully designed with demand imbalance and repositioning needs in mind. We develop a practical optimization framework for the problem using distributionally-robust optimization, to ensure robustness with respect to consumers’ uncertain adoption behavior. Calibrating the model to Car2go's operations data, we find that the car sharing service's profitability is highly sensitive to the choice of the service region. Furthermore, optimal deployment of free-floating car sharing could deliver significant environmental benefits by converting individually-owned cars into shared fleets of cleaner vehicles (e.g., EVs).
​
​
​
​
​
​
​
​
​
[8] L. He, H.Y. Mak, Y. Rong and Z.J.M. Shen (2017). Service Region Design for Urban Electric Vehicle Sharing Systems. Manufacturing & Service Operations Management, 19(2), 309-327.

Careful service region design is key to efficient free-floating car sharing operations [Credit: User:Wakasui/CC BY-SA 4.0]
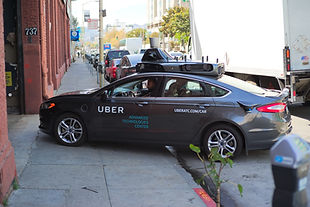
When self-driving cars become a reality, on-demand car sharing could dominate the mobility market in the future [Credit: User:Dllu/CC BY-SA 4.0]